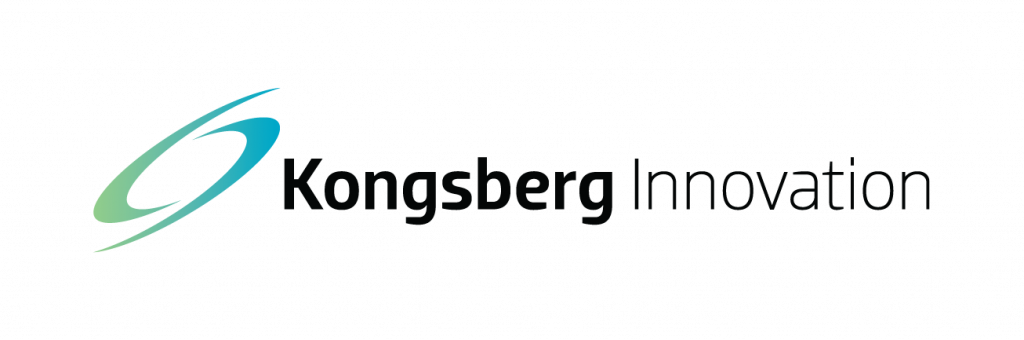
As a leading incubator for Norwegian deep-tech companies, we have shared insights in project meetings and workshops from a start-up perspective, and, importantly, learned from the other consortium members how they develop knowledge and methods for data-driven innovation. Additionally, in collaboration with a master student from USN, we explored the usage of (big) data in the early validation of startups. The research goal was to reduce the risk for everyone involved in the innovation ecosystem, including incubators and investors, and help high-tech startups use data to their advantage in the early stages, allowing them to validate their business ideas.
To explore the usage of data in early validation, we used a multi-case study research method to deeply understand the problem by selecting multiple startups and comparing them. This iterative approach allowed for better problem comprehension, efficient formulation of research questions, and realistic solutions. The research questions were initially formulated during a meeting with Kongsberg Innovation, guiding the literature search and adapting based on insights. Data was collected from semi-structured interviews with selected startups and a literature review, including peer-reviewed articles, conference papers, and grey literature. Three early-stage startups and one growth-stage startup were chosen as case studies due to their affiliation with KI and their high-tech business. The semi-structured interviews facilitated open discussions, helping to answer the research questions and create the user guide.
Our study explored the potential benefits of big data for early-stage high-tech startups using a market pull strategy in a B2B model. Our research suggests that startups often lack a structured validation approach, relying instead on direct stakeholder interactions. The startups face challenges such as limited data availability, resources, and short-term goals. We identified key factors impacting big data adaptation, including reluctance, lack of process, team experience, and constraints. To address these, an Early Validation User guide (EVU) based on lean and agile principles were developed to help startups integrate big data effectively during early validation and to decide when to pivot or scale.
Moreover, participation in H-SEIF 2 has significantly benefited Kongsberg Innovation by providing a deeper understanding of the types of projects, R&D challenges, and outcomes associated with data-driven product development among the industrial partners. The applicability of the methods developed in this project for startups, which typically have limited resources, remains to be explored. It is likely that a tailored simplification and customization to meet the specific needs of the startup segment will be necessary.